INTRODUCTION
Powerful machine learning (ML) image generators have recently become widely accessible to non-experts including artists, designers, and the general public, which has contributed to contentious discussions on the new aesthetics and creative practices that have emerged as a result. ML enables believable simulations to be produced based on statistical models trained on datasets of multitudes of other images. Generative ML systems have received a great deal of visibility for the affordances they offer, allowing realistic photographic images to be generated based on text inputs without significant technical knowledge. While images may be treated as the product of or interchangeable with data, the theoretical implications of this perspective are connected to longer tendencies in the history of photography and digital graphics, whereby technical modes of imaging have often been framed as observational rather than interpretive. Such perspectives on visual media are in need of critical reassessment as new methods expose a rift between speculative aspects entailed in imaging and the capacity for images to present visual knowledge (1). This research examines interdisciplinary practices working with data-intensive forms of visualisation in relation to existing theories on the epistemic qualities of visual technologies.
The widespread use of generative models to create images has resulted in particular aesthetic tendencies that often emulate the styles of other forms of visual media such as photography or painting while entailing significantly different processes and interpretive frameworks. This results in a discorrelation (2) between the subjective human perceptual interpretation of an image and the highly-automated technical systems that increasingly displace the importance of the latter. Although generative systems may create highly accurate visualisations based on data, the same techniques can also be used to produce images with little basis in reality. Synthetic images, for example, combine aspects of prior, observational approaches to imaging, with new generative methods, resulting in images whose accuracy is difficult to estimate. Not precisely scientifically objective, while also not being entirely fictional, synthetic images highlight the fact that the epistemic value of visual media is highly contextual. Considering these issues in relation to existing notions of scientific objectivity in visual media, this paper proposes a perspective on data-based aesthetics in which the production of images is understood as mediated through, rather than representative of data.
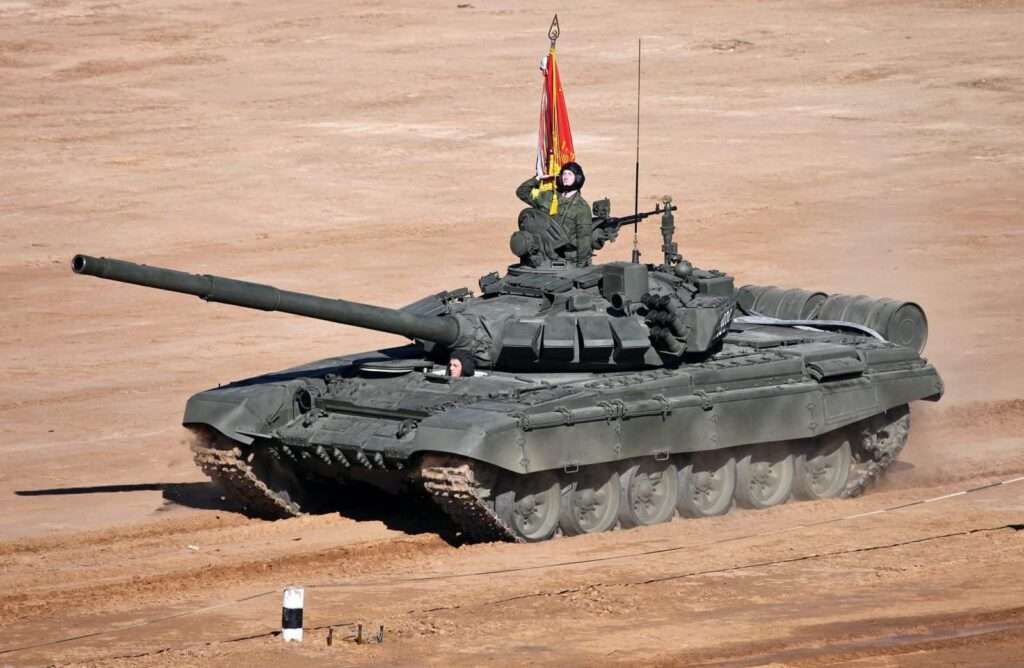
Figure 1: An image sourced online depicting a Russian T72-B3, via Forensic Architecture, 2018. Source: <http://content.forensic-architecture.org/wp-content/uploads/2019/09/spl1.jpg>

Figure 2: A Cinema 4D render of the same perspective of the tank in the previous image, Forensic Architecture, 2018. Source: <http://content.forensic-architecture.org/wp-content/uploads/2019/09/spl2.jpg>
SYNTHETIC IMAGES
Generative ML systems have facilitated the production of synthetic images (3) photorealistic digital renderings that can be used as training data for other ML systems. One way of thinking about this is that they are the product of synthesis rather than apparatus of capture, as in what are referred to in engineering contexts as real or natural images. But while this might seem like a straightforward distinction, it is less so on closer examination. For example, Goodfellow, Bengio, and Courville’s definition of a natural image hinges on the use of a camera:
“A natural image is an image that might be captured by a camera in a reasonably ordinary environment, as opposed to a synthetically rendered image, a screenshot of a webpage, etc.” (4)
While this is a functional definition, it doesn’t touch on a range of nuance that occurs between these categories of natural image and synthetic image, especially since digital photography is now the norm and it is increasingly commonplace for cameras to involve artificial intelligence (AI) features to enhance the images they produce. There is room for a great deal of variability in the qualities of images that have been captured by a camera. Conversely, a screenshot of a webpage can act as an accurate form of documentation of its visual content. The photographic paradigm of imaging, which is currently most dominant, also assumes a particular form of visual referentiality that is not always the case. Images are not necessarily representative of real-world objects and phenomena, and there are many aspects open to variability in the case of representational images.
Looking into this further, we can take insights from the work of Forensic Architecture, which often blurs the boundaries of fact and fiction. In many of their projects, the group aims to generate data that may be useable as a form of legally-recognised evidence, often for the purpose of tangibly proving disputed cases of human rights violations. Discussing their experimentation in the creation of synthetic data, Forensic Architecture explain:
“In order to train machine learning classifiers to detect a given object (the ‘object of interest’) in an image or video frame, a large dataset of images of the object of interest is usually necessary. Images of the objects of interest in the context of human rights violations (for example military vehicles, or chemical weapons) are often rare, however. Forensic Architecture has been experimenting with how to supplement a dataset of real images with additional ‘synthetic’ counterparts.” (5)
From this description, we understand that synthetic images arose in response to the problem of acquiring sufficient training data for a given kind of object. We may also note that there may be a reciprocal relationship between real and synthetic images, with the possibility for synthetic images to be used in place of real images. The figures above demonstrate this interrelationship, Fig. 1 depicting a Russian tank sourced from the internet, while Fig. 2 is a synthetic image of the same perspective of the tank in the previous image that has been generated by Forensic Architecture. Though they are fairly similar in appearance, there are subtle visual cues, such as resolution and detail, that perceptibly allow us to distinguish that one is more likely a real photograph, while the other has been generated. Yet, it’s easy to imagine situations where two such images could be visually indistinguishable. Indeed, Fig. 2 is an example of training data that has been generated, rather than gathered, but this leaves a lot of questions unanswered concerning the defining qualities of these two categories, especially given the fact that images sourced from the internet can have dubious truth value.
While images can, in practice, be used as data, for example, to train a model to produce other images, the relational aspects of synthetic images may render the distinction of real images merely positional within a given context, rather than being rooted in inherent qualities of the images, themselves. In this sense, synthetic images may subvert the forms of objectivity traditionally associated with the visual realism of optical media. For example, highly realistic images can be generated of virtually anything. In a well-known case, researchers demonstrated that it is possible to generate believable photographic images of people who do not exist (6). This is a case of simulacra, which Jean Baudrillard defines as copies without originals, describing simulation as “no longer that of a territory, a referential being, or a substance. It is the generation by models of a real without origin or reality: a hyperreal.” (7)
In generated images, or in this case, synthetic faces, each instance is a copy produced from a model of the human face. But no face is intended as a direct representation of an actual face that exists in the world. But another team of researchers then showed that this was not as straightforward as it initially seemed, in a paper entitled “This Face Does Not Exist … But It Might Be Yours!” (8), where the authors propose that the same system may also reproduce likenesses of real people from its training data. In this sense, it is difficult to say what such a generated image represents, lacking adherence to a specific real-world referent, while entailing the potential to replicate patterns in data with great accuracy.
ML tools can be used in scientific contexts to create highly accurate images, and in some cases this entails processes of visualizing the non-visual or the invisual (9). The relationship between the visual and processual aspects of images has been widely discussed in relation to the concept of the operational image, a term originally coined by Harun Farocki (10). He describes this kind of image as being defined by its performance of a spatial operation as opposed to its visual qualities as traditional perspectives on image-making.
An interesting case of this is the images of black holes produced by the event horizon telescope collaboration (11). In this case, a visualisation is made based on radio frequencies. The original phenomenon is non-visual in nature, meaning that we only have computational measurements to judge against, rather than being able to visually compare the representation against its referent. In another project called Cloud Studies by Forensic Architecture (12), visualisations are produced of cloud phenomena that are extremely ephemeral, and again, often non-visual. Using data visualisation techniques from gathered data about pollutants, or chemical weapons, they were able to track various environmental phenomena and create evidence of alleged criminal attacks that would be otherwise impossible to trace. Another growing area where ML has very promising applications is medical imaging and image analysis, where it can be used to help detect or even predict cancer, or to assist in surgeries.
But while there are cases where ML is able to create very accurate scientific images, a widely discussed aspect of ML is that it can also be subject to serious problems of inaccuracy and bias. This has been notably highlighted by researchers such as Joy Buolamwini, Timinit Gebru, Angelina McMillian-Major and Margaret Mitchell (13), who have pointed to its tendency towards further entrenching already existing inequalities including erring in ways that are deeply gendered, racist, homophobic, and classist. Beyond existing issues in the design, application, and management of ML systems, there is also an interpretive aspect of how we assess their outputs. For example applied to other contexts such as art and design, the resulting images are subject to different frameworks for analysis, interpretation, and evaluation than in technical and scientific research. The interrelationship between art and technoscientific methods, apparatuses, and aesthetics has a habit of destabilising the expectations we have about what the results of these processes mean. For example, in the work of artists who borrow or appropriate techniques from the sciences, the outcomes are then thrown into a new relationship with the world that is not governed by the same principles.
Aspects of the scientific worldview have been normalised to the point that they are taken as given, neutral, even. Artworks may be created using technical and scientific methods, but what they represent has little to do with scientific knowledge. They result, ultimately, in an aesthetic experience that uses the language of scientific imaging to communicate something else. There is therefore a need to develop methods, criteria and conceptual frameworks, such as those in art history and criticism, that allow us to grapple with what is entailed in such instances, where art coincides closely with science and technology.
Dan McQuillan proposes the approach of standpoint theory as a potential way forward from what he calls the necropolitics of AI, saying that “standpoint theory suggests the possibility of alternative ways knowing, rooted in the lived experience of people who are marginalized or minoritized. It is, first and foremost, a challenge, to the aura of absolute objectivity that places the scientific methods above other ways of knowing” (14). Artistic practices explore precisely these alternative ways of knowing that McQuillan describes, and I think critical explorations of synthetic images can help develop new frameworks for grasping them. For example, in the series Material Speculation of Moreshin Allahyari (Fig. 3) (15), the artist created 3d-printed models of cultural heritage destroyed by ISIS. Using what documentation remains of artworks that no longer exist, materially, the cultural memory of these artefacts is revived through the reinterpretation of known facts and concrete data such as Youtube videos of their destruction. Data sticks containing digital files for the 3d models the artist created are then embedded in the sculptures, in a direct sense combining the data with its spatial manifestation. In the process of reconstructing 3d models of these artefacts, Allahyari applies a speculative approach that is grounded in computational methods. The objects that are created through this process are both based in data, while also producing something new through the reinterpretation of the past, undermining the idea of data being self-evident or capable of standing in for its referent.
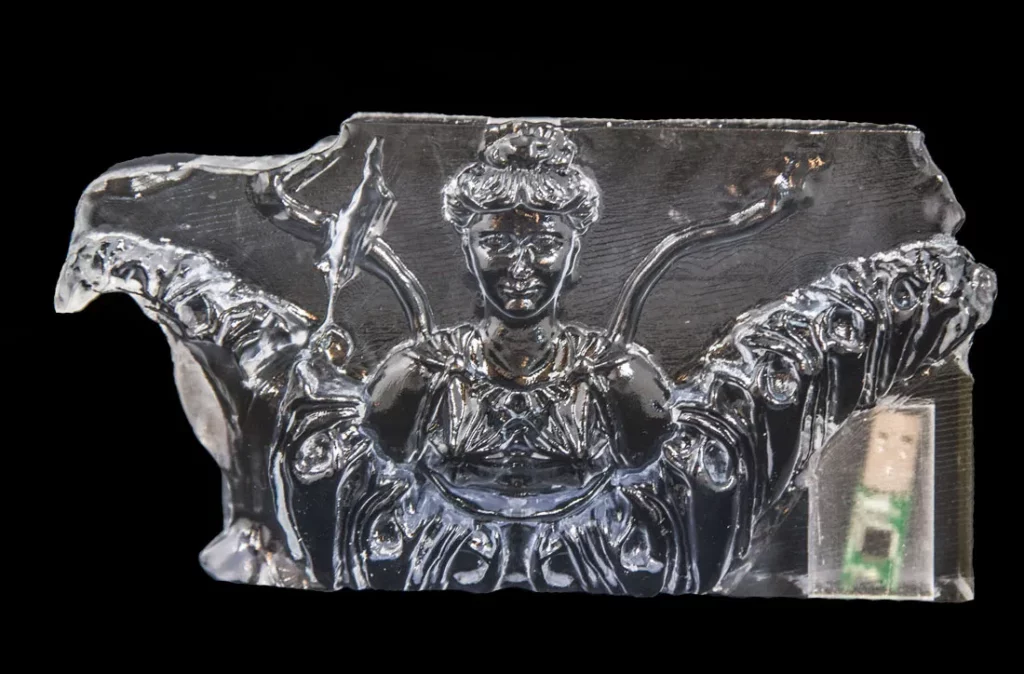
Figure 3: Material Speculation: ISIS: Marten, Morehshin Allahyari, 2016. © 2022 Morehshin Allahyari.
In a similar sense to the appropriation of technical and scientific methods outside of scientific contexts, synthetic images result in a divergence from prior conceptions of scientific objectivity in visual depictions, such as those outlined by Lorraine Daston and Peter Galison in their book Objectivity. They look specifically at conceptualisations of objectivity in scientific atlas images, from which they outline three main forms, or epistemic virtues (16). The first of these is truth-to-nature, referring to the verisimilitude or quality of being true-to-life in terms of visual referentiality. Botanical and biological illustration are good examples of this. A second form of objectivity is mechanical objectivity, in which direct physical mediation of a phenomenon occurs such as through photography or microscopy. The third form of objectivity Daston and Galison discuss is trained judgement, where the image does not aspire to visual realism, but rather involves a form of visual notation that must be interpreted in a particular, informed way. Importantly, these forms of objectivity are not mutually exclusive:
“Atlas images — whether reasoned, mechanical, or interpreted — bear the marks of both epistemology and ethos. [Objectivity] has traced how epistemology and ethos emerged and merged over time and in context, one epistemic virtue often in point-counterpoint opposition to the others. But although they may some times collide, epistemic virtues do not annihilate one another like rival armies. Rather, they accumulate: truth-to-nature, objectivity, and trained judgment are all still available as ways of image making and ways of life in the sciences today.” (17)
Something that Daston and Galison point out that draws an interesting parallel with machine learning is that these methods entail aspects of sample selection. Striving to present visual information in the most accurate way possible also involves choosing a sample that is deemed representative of the phenomenon depicted. This relationship between the many and the one involves the perfecting of nature, selecting an instance that can serve as an exemplary model of a given phenomenon. In that sense, these images strive to capture a generalisation in a specific instance, something that is echoed in the outputs of machine learning models. Lacking a steadfast relationship with direct observation, technical process, or trained judgement, recent visual practices such as creating images using generative models may mark a turn from representational towards what Daston and Galison refer to as presentational strategies of visual depiction:
“At this point, the relationship of science to aesthetics has departed from all our earlier models. Art and science are not self-evidently a single enterprise (few today assume that the True and the Beautiful must necessarily converge), nor do they stand in stalwart opposition to each other. Instead, they uneasily but productively reinforce each other in a few borderline areas.” (18)
The synthetic images generated by an ML system can be difficult to differentiate — aesthetically, computationally, and philosophically — from real or natural images. In this sense, they disrupt existing conceptual frameworks for technically-mediated vision, and give rise to new epistemic relationships. While generative image-making systems may be based on the large-scale analysis of data through highly technical methods, they are capable of producing outputs with variable degrees of accuracy.
DATA-BASED AESTHETICS
Discussing these ideas using the term data-based aesthetics invokes both the idea of an image being based on data, as in the synthetic images that are generated computationally from data, and the notion of aesthetics that present a form of visual knowledge. The view of images as the product of, interchangeable with, or as a form of data, in themselves, is connected to longer tendencies in the history of visual technologies, and it is important to reconsider how the epistemic basis of imaging may be reliant on problematic assumptions inherited from older imaging paradigms. Understanding media artifacts as data-based requires new perspectives that are informed by the processes and data behind the images, as opposed to viewing them in isolation.
Anticipating recent developments over fifteen years ago, Victoria Vesna addressed some of these issues in her book Database Aesthetics:
“In an age in which we are increasingly aware of ourselves as databases, identified by Social Security numbers and genetic structures, it is imperative that artists actively participate in how data are shaped, organized, and disseminated.” (19)
This perspective is a poignant reminder that while it may be commonplace to treat data as interchangeable with all things, the relational aspects of data are consequential, and aspects of the things that data stand in for are irreducible. Alva Noë notes, that “nothing is a model by virtue of its intrinsic makeup alone.” (20) Models are ways of exploring the world or accomplishing certain goals. A model is useful, successful, accurate only insofar as it achieves a purpose. So ultimately, it is not the intrinsic qualities of the model that define it as such. In this sense, the measure of a model is its functionality or efficiency at achieving whatever purpose it is applied to. The danger of considering data-based images as separate from the models and worldviews they emerge from would be to neutralise the particular position they derive from.
Like data, models are relational in nature, and this can meaningfully inform our perspective on visual media. One aspect of this is that data don’t tell us much in themselves, and they are always subject to interpretation. This idea is informed by the view of postphenomenolgy (21), which asserts that the mediation of perceptual experience sets up hermeneutic relationships in which what is tangible of the original phenomenon is interpreted and altered in the process. As Daston and Galison describe, recent forms of data-based imaging may constitute a fusing of the artifactual with the natural (22), a form of epistemic practice that produces visual knowledge.
In Johanna Drucker’s work on visual epistemology and interpretive digital tools, she says “The field of visual epistemology draws on an alternative history of images produced primarily to serve as expressions of knowledge.” (23) It holds a base assumption that knowledge may be expressed in visual form, which is something that is often questioned with regard to art. Drucker goes on to explain that the interpretation of visualisations is intimately entangled with the visual interpretation of information, highlighting the contextual and interpretive nature of data. The aesthetic, conceptual and methodological approach applied in image-making may inform the way images are in turn interpreted, and this is further complicated when we add in a basis in data.
Aurora Hoel proposes the operationally real as an alternative to dichotomies typically applied to separate the real from the virtual or the unreal:
“The operationally real is an intermediate reality instituted and sustained by the mediating or resolving action of some technical apparatus (e.g., a living body or a technical machine) that plays the role of adaptive mediator. This implies that the operationally real is a mixed environment of a middle order of magnitude—a complex milieu made up of heterogeneous and conflicting elements and powers that have been brought into communication by technicity.” (24)
In the sense that synthetic images may perform the function of real images, in training an ML system and to be included in their training data, these images may be considered to be operationally real. As Hoel puts it, “The operationally real is a mixed reality, a virtuality instituted and sustained by mediation.” (25) To think of data-based imaging practices from this perspective enables us to acknowledge the positional and relational aspects of data and visualisations based on it, as opposed to attempting to divide these into separate categories. Just as Noë pointed out about models not being defined by their intrinsic makeup, but rather the goals they are applied to, so to can this apply to the data basis of images.
CONCLUSION
In comparison to previous paradigms of image-making, recent technical developments entail complex relationships between the perceptible and its interpretation. The processes behind an image often matter to its interpretation, even if they are not visually accessible within the image. And our understandings of technology inform the expectations we have for interpreting these relationships between the visual and the processual. Consequently, highly technical forms of visual media may require more knowledge external to the image itself, reshaping the way we visually decipher images but also how we manage their contextualisation within larger frameworks beyond the strictly visual.
The ways in which AI influences visual mediatation can be difficult to predict, evaluate, remediate, or resist. This underscores the need for perspectives that may challenge the dominant narratives around AI. With the magnitude of visual data that are produced, circulated, and analysed on a daily basis, it is increasingly important to consider alternative perspectives, such as those proposed by standpoint theory, visual epistemology, or the view that there are multiple forms of visual objectivity. Ultimately, the idea of the image as the product of or a form of data itself is one that is incredibly nuanced and it requires us to develop new modes of critical enquiry in order to grasp emerging, data-based aesthetics.
REFERENCES
1. Johanna Drucker, Visualization and Interpretation: Humanistic Approaches to Display (Cambridge: MIT Press, 2020).
BACK
2. Shane Denson, Discorrelated Images (Durham: Duke University Press, 2020).
BACK
3. Forensic Architecture, Experiments in Synthetic Data (2018) <forensic-architecture.org/investigation/experiments-in-synthetic-data>, accessed November 2023.
BACK
4. I Goodfellow, Y Bengio and A Courville, Deep Learning (Cambridge: MIT Press, 2016).
BACK
5. Forensic Architecture (2018). [3.]
BACK
6. T Karras, S Laine and T Aila, “A Style-Based Generator Architecture for Generative Adversarial Networks”, Computer Vision and Pattern Recognition Conference Proceedings, pp. 4401-4410 (2019).
BACK
7. Jean Baudrillard, Simulacra and Simulation (Ann Arbor: Univ. Michigan Press, 1981).
BACK
8. P Tinsey, A Czajka, and P Flynn, “This Face Does Not Exist … But It Might Be Yours! Identity Leakage in Generative Models”, arXiv:2101.05084 [cs.CV] (2020).
BACK
9. Jussi Parikka, Operational Images: From the Visual to the Invisual (Minneapolis: University of Minnesota Press, 2023).
BACK
10. Harun Farocki, “Phantom Images”, Public 29, pp. 12–22 (2004).
BACK
11. Event Horizon Telescope Collaboration, “First M87 Event Horizon Telescope Results. I. The Shadow of the Supermassive Black Hole”, The Astrophysical Journal Letters 875, No. 1 (2019).
BACK
12. Forensic Architecture, Cloud Studies (2020) <https://forensic-architecture.org/investigation/cloudstudies>, accessed November 2023.
BACK
13. E Bender, T Gebru, A McMillian-Major and S Shmitchell, “On the Dangers of Stochastic Parrots: Can Language Models Be Too Big?”, FAccT ’21 – ACM Conference on Fairness, Accountability, and Transparency Proceedings, pp. 610–623 (2021).
BACK
14. Dan MacQuillan, Resisting AI (Bristol: Bristol University Press, 2022) p. 105.
BACK
15. Moreshin Allahyari, Material Speculation: ISIS: Marten (2016) <https://morehshin.com/material-speculation-isis>, accessed November 2023.
BACK
16. Lorraine Daston and Peter Galison, Objectivity (New York, Cambridge: Zone Books, 2007) pp. 39–41.
BACK
17. Daston and Galison, p. 363. [16.]
BACK
18. Ibid., p. 412.
BACK
19. Victoria Vesna, Database Aesthetics: Art in the Age of Information Overflow (Minneapolis: University of Minnesota Press, 2007) p. xiii.
BACK
20. Alva Noë, Strange Tools: Art and Human Nature (New York: Hill and Wang, 2015) p. 153.
BACK
21. Don Ihde, “Introduction: Postphenomenology”, in Postphenomenology: Essays in the Postmodern Context (Evanston: Northwestern Univ. Press, 1993) pp. 1–8.
BACK
22. Daston and Galison, p. 413. [16.]
BACK
23. Drucker, p. 17. [1.]
BACK
24. AS Aurora Hoel, “Technicity and the Virtual”, Humanities 11, No. 6 (2022) p. 18.
BACK
25. Hoel, p. 20. [24.]
BACK